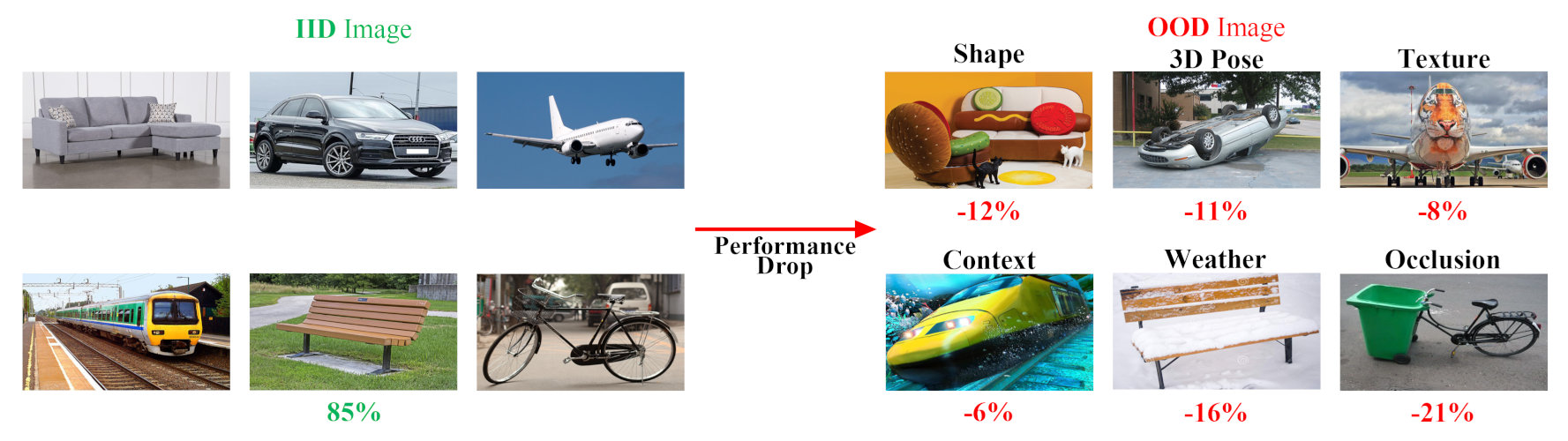
Deep learning models are usually developed and tested under the implicit assumption that the training and test data are drawn independently and identically distributed (IID) from the same distribution. Overlooking out-of-distribution (OOD) images can result in poor performance in unseen or adverse viewing conditions, which is common in real-world scenarios.
In this workshop, we are interested in discussing the performance of computer vision models on OOD images which follows a different distribution than the training images. Also given the current trend of web-scale pretrained computer vision models, it is of interest to better understand their performance in the OOD or rare scenarios.
Our workshop will be featuring three competitions, OOD generalization on the OOD-CV dataset, open-set recognition and generalized category discovery on the Semantic-shift benchmark.